12 April 2024
Unlocking Agricultural Insights with Satellite Data and Machine Learning Algorithms
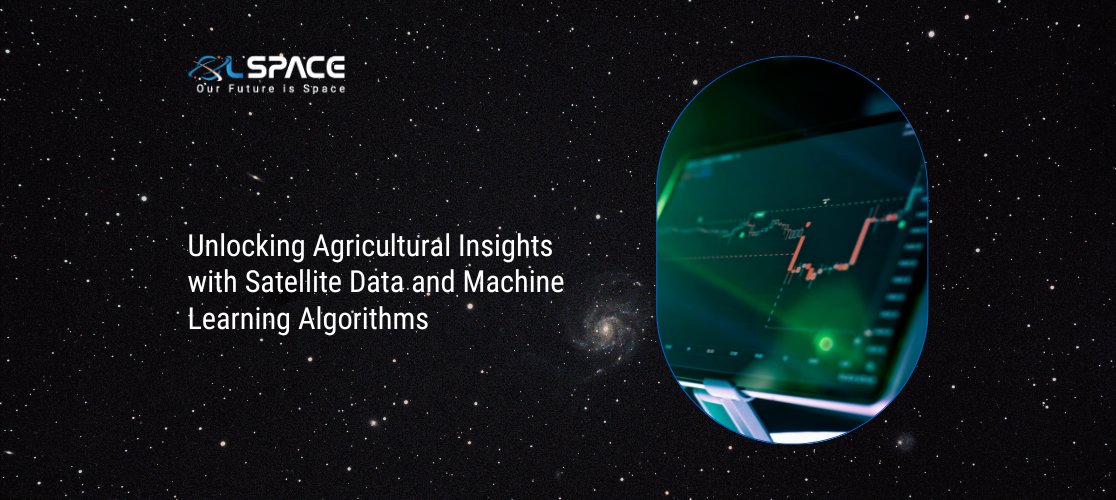
The convergence of satellite data and machine learning algorithms has revolutionised agricultural practices by providing farmers, researchers, and policymakers with unprecedented insights into crop health, yield prediction, and resource management. By harnessing the power of remote sensing technology and advanced analytics, agricultural stakeholders can optimise decision-making processes, increase productivity, and promote sustainable farming practices. This article explores how the integration of satellite data and machine learning algorithms unlocks valuable insights for agricultural applications.
1. Remote Sensing Technology in Agriculture:
Remote sensing technology, facilitated by satellites orbiting the Earth, captures multispectral imagery and other data related to land cover, vegetation health, soil moisture, and weather patterns. These data layers offer a comprehensive view of agricultural landscapes, allowing for the monitoring of crop growth, pest infestations, and environmental conditions with spatial and temporal granularity.
2. Machine Learning Algorithms for Data Analysis:
Machine learning algorithms, including supervised and unsupervised techniques such as neural networks, random forests, and support vector machines, process large volumes of satellite data to extract meaningful insights. These algorithms learn patterns and relationships within the data, enabling tasks such as classification, regression, anomaly detection, and clustering.
3. Crop Health Monitoring and Disease Detection:
Satellite data combined with machine learning algorithms enable the monitoring of crop health and the early detection of diseases and pests. By analysing spectral signatures and vegetation indices derived from satellite imagery, algorithms can identify anomalies indicative of stress, nutrient deficiencies, or pathogen infestations, allowing farmers to take timely corrective actions.
4. Yield Prediction and Crop Monitoring:
Machine learning models trained on historical satellite data and ground truth observations can predict crop yields and monitor crop growth throughout the growing season. By analysing factors such as vegetation indices, weather patterns, and soil characteristics, these models provide accurate yield forecasts, helping farmers optimise input usage, harvest timing, and marketing strategies.
5. Precision Agriculture and Resource Management:
Satellite data and machine learning algorithms support precision agriculture practices by guiding targeted interventions and resource allocations. By identifying variability within fields, optimising irrigation schedules, and prescribing site-specific treatments, farmers can minimise input costs, reduce environmental impact, and maximise crop yields on a per-acre basis.
6. Climate Resilience and Adaptation Strategies:
Agricultural stakeholders use satellite data and machine learning algorithms to assess climate risks and develop adaptation strategies in response to changing environmental conditions. By monitoring temperature extremes, precipitation patterns, and drought indices, algorithms provide early warnings of climatic hazards, enabling farmers to implement resilience measures and diversify cropping systems accordingly.
7. Policy Support and Decision-Making:
Governments and agricultural organisations leverage satellite data and machine learning insights to inform policy decisions, allocate resources, and monitor compliance with agricultural regulations. By assessing land use changes, monitoring deforestation rates, and evaluating ecosystem services, policymakers can design evidence-based interventions to promote sustainable land management and biodiversity conservation.
8. Challenges and Future Directions:
While the integration of satellite data and machine learning offers immense potential for agricultural innovation, challenges such as data interoperability, algorithm scalability, and model interpretability remain to be addressed. Continued research, investment in infrastructure, and stakeholder collaboration are essential to realising the full benefits of this technology for agricultural sustainability and food security.
Conclusion:
The synergy between satellite data and machine learning algorithms empowers agricultural stakeholders with actionable insights to address complex challenges and capitalise on emerging opportunities in the agricultural sector. By leveraging these technologies, farmers can optimise resource use, mitigate risks, and enhance productivity in a rapidly changing environment. As advancements in remote sensing and data analytics continue to unfold, satellite-based agricultural monitoring and decision support systems will play an increasingly pivotal role in shaping the future of global food systems and sustainable development.