26 March 2024
The Power of Predictive Modeling: How AI and Big Data Improve Disaster Forecasting
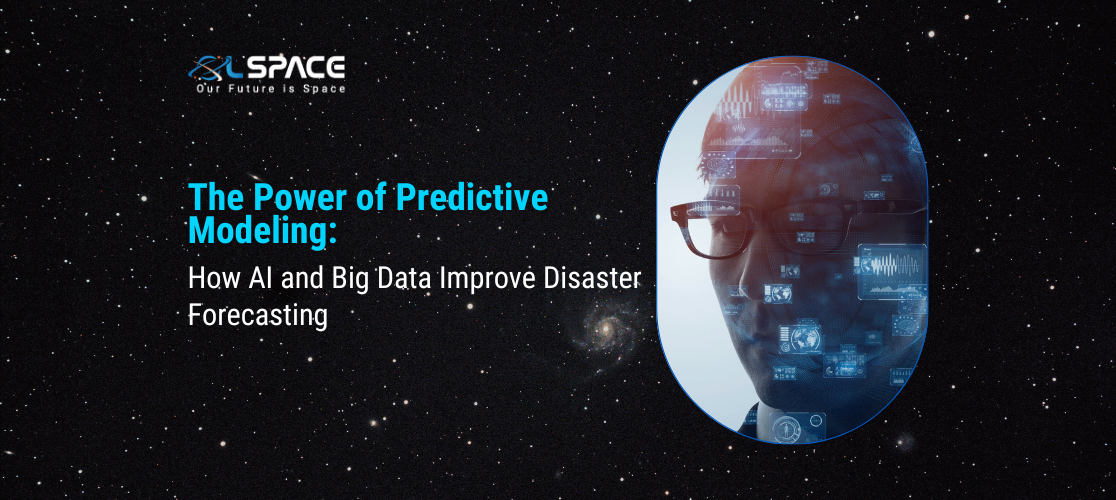
Predictive modeling, powered by artificial intelligence (AI) and big data analytics, has revolutionised disaster forecasting and management. By leveraging vast amounts of data and advanced algorithms, predictive models can provide early warnings, assess risks, and guide proactive measures to mitigate the impact of natural disasters. This article explores the transformative role of AI and big data in improving disaster forecasting and response strategies.
Harnessing Big Data:
Big data encompasses a wide range of sources, including satellite imagery, weather data, seismic data, historical disaster records, social media feeds, and sensor networks. By aggregating and analyzing these diverse datasets, predictive models gain insights into environmental patterns, trends, and potential risk factors.
AI Algorithms for Prediction:
AI algorithms such as machine learning and deep learning excel in pattern recognition, anomaly detection, and predictive analytics. In disaster forecasting, these algorithms can learn from historical data, identify correlations, and generate forecasts based on probabilistic models and scenarios.
Early Warning Systems:
Predictive modeling enables the development of early warning systems for various disasters such as hurricanes, floods, wildfires, earthquakes, and tsunamis. These systems analyze real-time data streams, detect anomalies or precursor events, and issue timely alerts to authorities and communities at risk, allowing for proactive evacuation and preparedness measures.
Risk Assessment and Vulnerability Mapping:
AI-driven predictive models assess risk levels and vulnerabilities in specific regions or infrastructure. By considering factors like terrain, climate patterns, population density, infrastructure resilience, and historical disaster data, these models can prioritise areas for mitigation efforts, infrastructure improvements, and disaster risk reduction strategies.
Dynamic Modeling and Scenario Planning:
AI-powered models can simulate dynamic scenarios and assess the potential impact of disasters under different conditions. This capability is valuable for emergency planning, resource allocation, evacuation route optimisation, and decision-making during crisis situations, ensuring preparedness for varying intensities or trajectories of disasters.
Real-Time Monitoring and Response:
Integrating AI and big data analytics into disaster response systems enables real-time monitoring, situational awareness, and adaptive responses. Automated systems can analyze incoming data streams, detect changes or anomalies, coordinate emergency responses, allocate resources efficiently, and provide decision support to responders and policymakers.
Community Engagement and Communication:
Predictive modeling also supports community engagement and risk communication efforts. By visualising risk maps, probabilistic forecasts, and impact scenarios, communities can better understand their vulnerability, participate in preparedness activities, and make informed decisions to protect lives and property.
Continuous Improvement and Learning:
I-driven predictive models continuously learn and improve over time as they ingest new data and validate forecasts against actual events. This iterative process of model refinement enhances the accuracy, reliability, and effectiveness of disaster forecasting and response strategies.
Conclusion:
The combination of AI and big data analytics has unleashed the power of predictive modeling in disaster forecasting and management. These technologies enable early warnings, risk assessments, scenario planning, real-time monitoring, and adaptive responses that enhance resilience and save lives during natural disasters. Embracing AI-driven predictive modeling represents a significant leap forward in disaster preparedness, response capabilities, and community resilience, paving the way for a safer and more resilient future in the face of environmental challenges.